

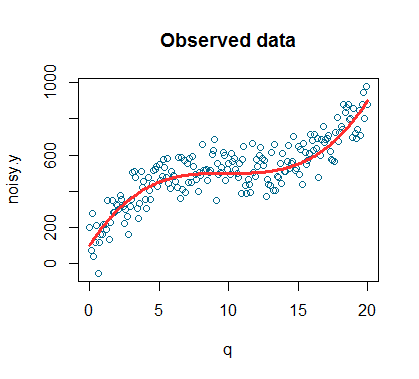
Compute increase in R2 from sum of square difference.Compare sum of squares between models from ANOVA results.Check F-statistics and p-values for the SS differences.Compute difference in sum of squares for each step.Build sequential (nested) regression models by adding variables at each step.The formal steps that we will following to complete this analysis is as follows. In this complex research question we essentially want to know if adding advertising cost will improve the model significantly in terms of the r square. Is a model that explains the total sales of an object with Urban location, US location, shelf location, price, income and advertising cost as independent variables superior in terms of R2 compared to a model that explains total sales with Urban location, US location, shelf location, price and income as independent variables? Another way to see this is in the following research question For example, if a previous model was able to predict the total sales of an object using three variables you may want to see if a new additional variable you have in mind may improve model performance. Often the primary goal with hierarchical regression is to show that the addition of a new variable builds or improves upon a previous model in a statistically significant way. Model 4 = Sales~Urban + US + ShelveLoc + price + income + Advertising Model 3 = Sales~Urban + US + ShelveLoc + price + income Our goal will be to predict total sales using the following independent variables in three different models. We are going to use the “Carseats” dataset from the ISLR package. This approach is a model comparison approach and not necessarily a statistical one. Hierarchical regression analysis is used in situation in which you want to see if adding additional variables to your model will significantly change the r2 when accounting for the other variables in the model.
REGRESSION IN R STUDIO HOW TO
In this post, we will learn how to conduct a hierarchical regression analysis in R.
